Realizing a Sustainable Mobility Society with Traffic Flow Data IHI is contributing to the early realization of a safe, secure mobility society by using traffic flow data generated with IHI’s LiDAR technology
IHI Corporation
A new mobility society is coming. It is represented by trends such as MaaS (Mobility as a Service) and CASE (Connected, Autonomous, Shared, and Electric). IHI, whose 3D Laser Radar system improves traffic safety, is envisioning the near-future mobility society. This article describes IHI’s endeavors to realize this new society.
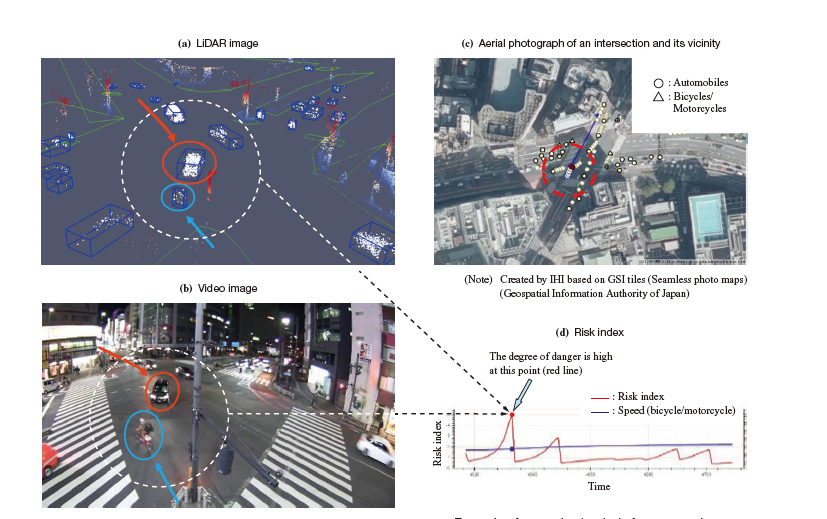
The future of the mobility society
Autonomous driving technologies are in active development all over the world. These technologies are closely related to MaaS and referred to as examples of CASE. Various projects are being planned and carried out around the world, such as automated taxicabs, automated buses, and automated valet parking services as well as automated delivery services and unmanned convoy traveling of large trucks in the logistics industry. These days, in addition to the development of automated vehicles in themselves, autonomous driving technologies for improving safety and convenience are being developed in combination with sensor systems installed as road infrastructure facilities. IHI has developed a traffic safety support system using LiDAR (Light Detection And Ranging) devices and applied this system to various places. This article describes our endeavors to realize the near-future mobility society that we envision based on our ever-accumulated technology.
IHI’s endeavors made thus far
IHI began the development of sensing technologies to support the automation of transport machines and heavy machines in adverse environments in the 1990s and developed 3D Laser Radar, the original LiDAR sensor for recognizing objects three-dimensionally. This system has been employed as a level-crossing obstacle detection system for railroads. We have delivered more than 2 400 systems in Japan, Europe and other areas since 2005, when we delivered the first system. This system facilitates safe rail operation by detecting automobiles and pedestrians stranded at railroad crossings and sending such information to the crew of approaching trains to prevent accidents. In addition, we are also applying this system to the area of ITS (Intelligent Transport Systems). Unlike the level-crossing obstacle detection system, which detects objects that remain at railroad crossings, this application requires to track and categorize moving objects such as automobiles and trucks. We have established these more advanced detection processing technologies by performing various tests. The 3D Laser Radar is adopted for the DSSS (Driving Safety Support System) by the UTMS (Universal Traffic Management Systems) Society of Japan’s support system, thus contributing to traffic safety. In addition, we have carried out demonstration experiments in Singapore to further improve this system (refer to “Monitoring Intersections to Alert Drivers” IHI Engineering Review, Vol. 49, No. 2, 2016).
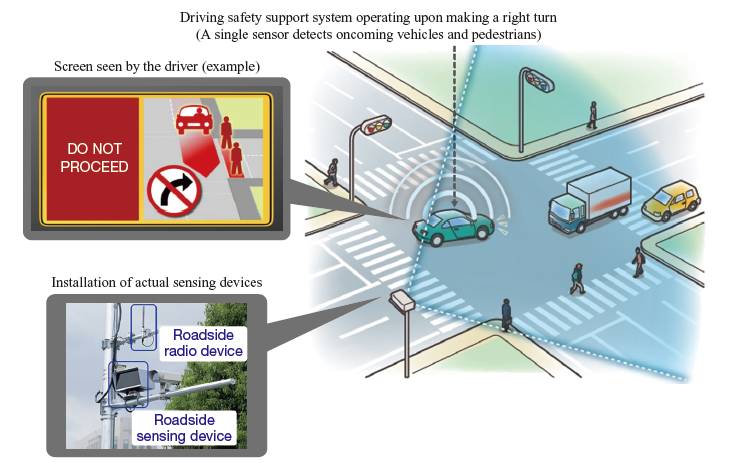
In recent years, we are participating in autonomous driving bus demonstration projects that are now being conducted in various locations throughout Japan. We are demonstrating support for safe bus operation by sending information on oncoming vehicles to autonomous driving buses at low-visibility intersections without traffic lights on their routes.
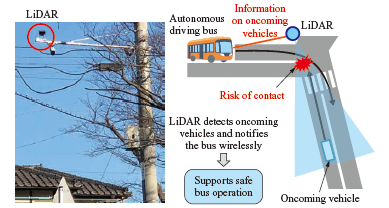
Issues to be solved to realize autonomous driving
As the development of autonomous driving systems speeds up in the automobile industry, their actual application is expected to be realized earlier. However, one issue in the development of autonomous driving systems is that the test case data obtained on actual public roads that is applicable to verification and simulation is still insufficient. For this reason, it is difficult to ensure safety, which is essential to establish autonomous driving systems. In order to verify the safety of autonomous driving systems under development, it is important to obtain test case data that covers every possible traffic scenario on public roads, including accidents and near-miss incidents. However, there is insufficient information on the processes leading to accidents and the surrounding circumstances other than the people involved in the accidents, because currently it is customary for test case data to be prepared manually based on each traffic accident report. The point at issue is the lack of completeness of information necessary to verify the functional safety of autonomous driving systems.
Therefore, a traffic measurement method to obtain data on the actual traffic situation comprehensively is necessary. To this end, video cameras are generally used. However, because the images recorded by such cameras are two dimensional, the data has low accuracy in terms of the relative positions in the depth direction between traffic participants such as automobiles, motorcycles, bicycles, and pedestrians, which makes it hard to extract traffic scenarios. Another difficulty is that video camera images are susceptible to their environments because the amount of ambient light varies according to the time of day and weather. As an alternate method in Japan, travel history information provided by the ETC2.0 equipment installed in each vehicle can be utilized. However, its positional information is not currently accurate enough and the equipment is not widely used. On the other hand, the LiDAR system can obtain positional information of traffic participants more precisely than video cameras without being influenced by the environment because the system with the time-of-flight method emits light toward objects and detects the reflected light in order to measure the distances to such objects. Utilizing LiDAR to measure traffic flows and obtain objects’ positional information has enabled us to extract traffic scenarios and generate/simulate the test case data necessary to develop autonomous driving systems.
Generation of accurate object sizes and trajectories
Because the precise moving trajectories of traffic participants are required to prepare test case data, IHI has developed various technologies.
For example, in the case that only one LiDAR unit is installed on a general public road, when a large truck or bus passes through the space in front of the LiDAR unit, its front view is obstructed as is the case for people’s sight. In such a case, the laser light emitted from the LiDAR unit irradiates the surface of the object ahead, and only this object can be detected (occurrence of a blind spot), making it difficult to know the traffic situation on the opposite side of the object. IHI sets up multiple LiDAR units to prevent generation of such blind spots and compiles all the data obtained by all LiDAR units into a single set with technologies for LiDAR calibration and for consistently integrating the time series data from the multiple LiDAR units. This enables the generation of accurate sizes and moving trajectories of running vehicles. Even the trajectories generated in this way contain deviations and missing data due to measurement errors. In response to this problem, we applied an algorithm that estimates and smooths trajectories, thus enabling the generation of corrected trajectories with high moving precision.
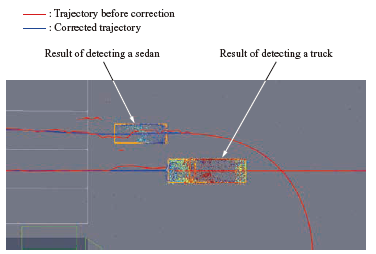
Extraction of near-miss cases
Using the above-mentioned high-precision moving trajectories, we have developed a data processing technology to extract near-miss cases, which are potential traffic accidents.
In spite of the smallness in number of recorded near-miss cases, we believe that these cases, which nearly become accidents, are necessary to improve the completeness of the test case data for developing autonomous driving systems. Therefore, IHI developed a data processing technology that extracts cases considered to be near-miss cases by defining the danger degree parameter (risk index) using traffic participants’ moving speeds and degrees of closeness from the generated moving trajectory data. The following figure shows an example that extracts a near-miss case involving a right-turn vehicle and a bicycle. This technology can be used to extract specific near-miss cases from big data, which can be used as test case data to develop autonomous driving systems.
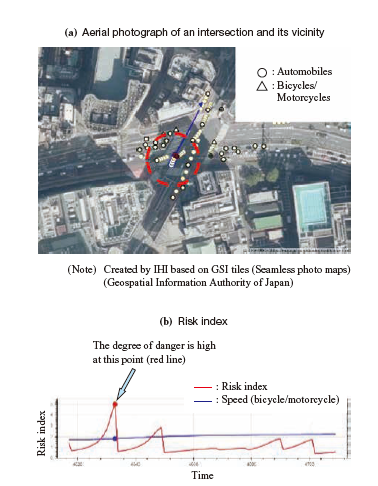
Application to traffic simulation
Toward the realization of a safe, secure mobility society, in parallel with the development of autonomous driving systems, it is also important to improve road environments (to reduce traffic accidents and traffic jams). These are recognized as issues around the world, and in Japan as well, there are intersections all over the country at which traffic accidents and traffic jams frequently occur. Although video cameras are generally used to analyze the traffic conditions at such intersections, it is not easy to obtain accurate relative positions among traffic participants. In addition, a traffic simulator can be used as a method for verifying traffic jams. However, to improve simulation accuracy, input parameters must be close to those of the actual movements. Analyzing LiDAR data can improve traffic simulation accuracy because it not only captures the conditions of traffic jams and accidents just like video data can but also obtains traffic participants’ moving speeds and acceleration data.
Traffic simulators can simulate traffic conditions and the effects of traffic light control, which is useful for verifying road improvements to reduce traffic jams. However, actual road environments include numerous combination patterns of road conditions, such as varied vehicle types, drivers’ driving characteristics, road shapes, weather conditions, and time of day. For this reason, it is extremely difficult to reproduce actual movements in a simulator. Therefore, we have been developing a technology that extracts parameters from actual traffic flow data, including traffic participants’ moving speeds and acceleration generated from LiDAR data, and inputs these parameters into a traffic simulator. This technology enables high-precision verification in reference to the data of actual scenes on public roads and contributes to improving road environments using digital twins.
Toward the realization of a safe, secure mobility society
HI is making efforts to resolve issues toward the early realization of autonomous driving systems by utilizing various traffic flow data obtained by the LiDAR system. We will extract the features of each local area from the traffic flow data obtained in the respective areas, and we will combine LiDAR data with various data and simulation technologies in order to generate “moving towns” in virtual space — in other words, to realize digital twin towns. Inside this virtual space, pedestrians and automobiles move around similarly to the real world, and near-miss incidents and traffic jams can occur. Continuously simulating such conditions and making predictions about them enables us to take measures to prevent accidents and to reduce traffic jams in the real world. We believe these “moving towns” will lead to the realization of true smart cities in which data utilization harmonizes safety and mobility as well as reduces environmental loads due to traffic jams.
In this way, IHI will contribute to achieving safe, secure, and easy-to-live-in towns using LiDAR technologies and realizing a sustainable mobility society.